Learning Spatial Relations
Mateusz Malinowski and Mario Fritz
Abstract
Over the last two decades we have witnessed strong progress on modeling visual object classes, scenes and attributes that have significantly contributed to automated image understanding. On the other hand, surprisingly little progress has been made on incorporating a spatial representation and reasoning in the inference process. In this work, we propose a pooling interpretation of spatial relations and show how it improves image retrieval and annotations tasks involving spatial language. Due to the complexity of the spatial language, we argue for a learning-based approach that acquires a representation of spatial relations by learning parameters of the pooling operator. We show improvements on previous work on two datasets and two different tasks as well as provide additional insights on a new dataset with an explicit focus on spatial relations.
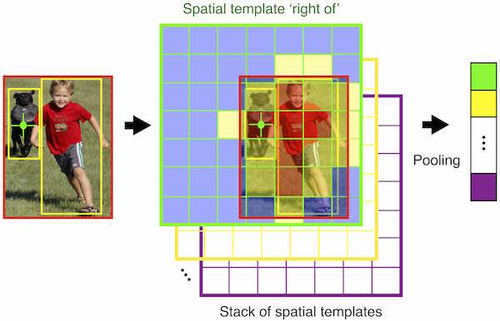
Dataset of Structured Queries and Spatial Relations
We have annotated SUN09 dataset with structured queries using a human notion of spatial relations (object-spatial relation-object).
If you use our dataset, please cite:
@article {mmalinowski14spatialpooling,
title = {A Pooling Approach to Modelling Spatial Relations for Image Retrieval and Annotation},
journal = {arXiv:1411.5190 [cs.CV]},
year = {2014},
month = {November},
url = {http://arxiv.org/abs/1411.5190},
author = {Mateusz Malinowski and Mario Fritz}
}
References
[1] A Pooling Approach to Modelling Spatial Relations for Image Retrieval and Annotation. M. Malinowski, and M. Fritz. arXiv 2014.