Articulated People Detection and Pose Estimation
In this work we are interested in the challenging problem of articulated people detection pose and estimation in real-world sport scenes. State-of-the-art methods for human detection and pose estimation require many training samples for best performance. While large, manually collected datasets exist, the captured variations w.r.t. appearance, shape and pose are often uncontrolled thus limiting the overall performance. In order to overcome this limitation we propose a new technique [1] to extend an existing training set that allows to explicitly control pose and shape variations. For this we build on recent advances in computer graphics to generate samples with realistic appearance and background while modifying body shape and pose.
Data generation method [1]
Below is the overview of our novel data generation method consisting of three stage
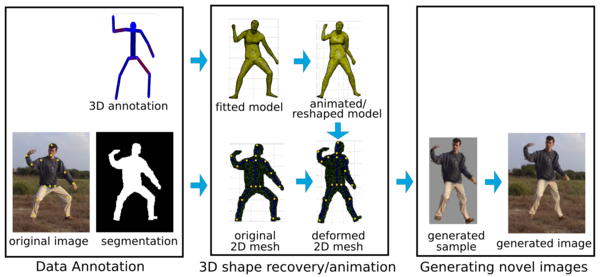
Starting from approximate 3D pose annotations we first recover the parameters of the 3D human shape model. The body shape is then modified by reshaping and animating. Reshaping changes the shape parameters according to the learned generative 3D human shape model and animating changes the underlying body skeleton. Given the new reshaped and/or animated 3D body shape we backproject it into the image and morph the segmentation of the person. To that end we employ the linear blend skinning procedure with bounded biharmonic weights. After pose and shape changes are applied we render the appearance to the deformed 2D segmentation and backproject the novel sample into the background image.
Examples of novel training samples
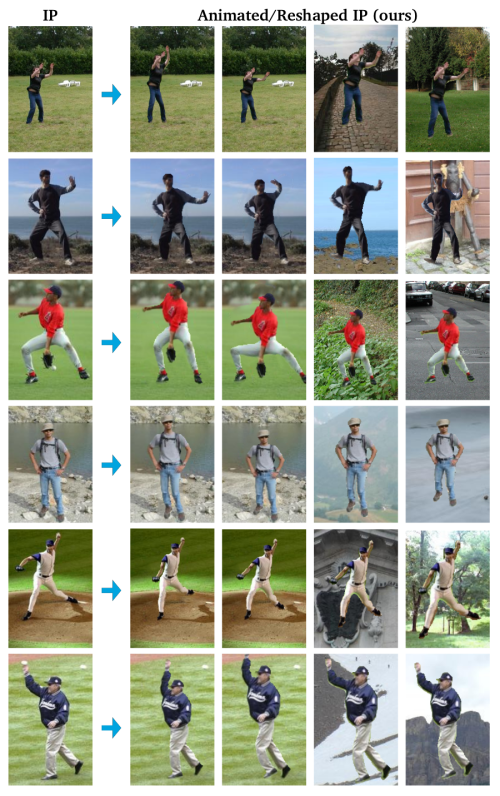
Shown are original images from the "Image Parsing" (IP) dataset (left) and novel samples obtained by reshaping and animation (right).
The data is available here.
Sample results on IP dataset
We validate the effectiveness of our approach on the task of articulated human detection and articulated pose estimation. We report close to state of the art results on the popular "Image Parsing" (IP) human pose estimation benchmark and demonstrate superior performance for articulated human detection.
Sample detections on IP dataset by DPM trained on our synthetic and real data, and on real data alone are shown below.
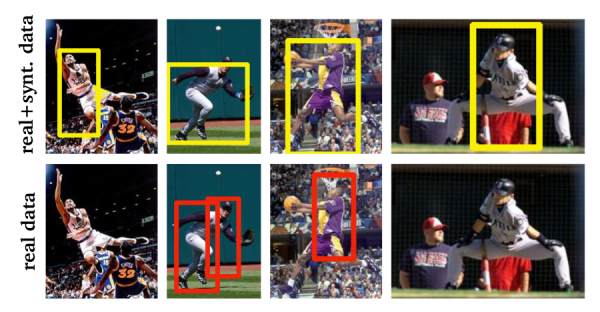
We also provide sample pose estimation results obtained by our novel Joint PS+DPM model which integrates the evidence from the DPM into the Pictorial Structures (PS), and compare to the results obtained by PS model.
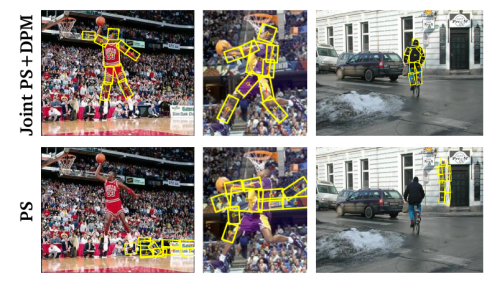
Articulated pose estimation "in the wild"
We define a new dataset based on the "Leeds Sport Poses" (LSP) dataset by using the publicly available original non-cropped images. This dataset which we denote as "Multi-scale LSP", contains 1000 images depicting multiple people in different poses and at various scales. Below are shown sample images from the new dataset together with detections and poses estimations obtained by our method.
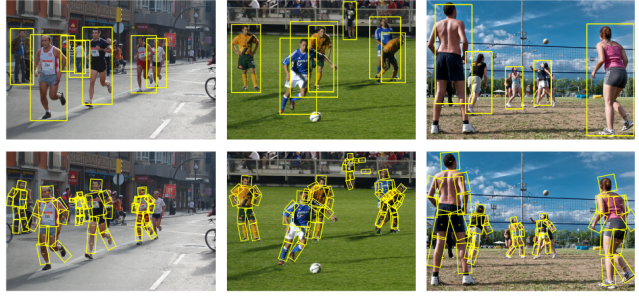
The annotations are available here.
Poster
References
[1] Articulated People Detection and Pose Estimation: Reshaping the Future, L. Pishchulin, A. Jain, M. Andriluka, T. Thormaehlen and B. Schiele, IEEE Conference on Computer Vision and Pattern Recognition (CVPR), June, (2012)