Multi-Class Video Co-Segmentation
Wei-Chen Chiu and Mario Fritz
Abstract:
Video data provides a rich source of information that is available to us today in large quantities e.g. from online resources. Tasks like segmentation benefit greatly from the analysis of spatio-temporal motion patterns in videos and recent advances in video segmentation has shown great progress in exploiting these addition cues. However, observing a single video is often not enough to predict meaningful segmentations and inference across videos becomes necessary in order to predict segmentations that are consistent with objects classes. Therefore the task of video co-segmentation is being proposed, that aims at inferring segmentation from multiple videos. But current approaches are limited to only considering binary foreground/background segmentation and multiple videos of the same object. This is a clear mismatch to the challenges that we are facing with videos from online resources or consumer videos.
We propose to study multi-class video co-segmentation where the number of object classes is unknown as well as the number of instances in each frame and video. We achieve this by formulating a non-parametric bayesian model across videos sequences that is based on a new videos segmentation prior as well as a global appearance model that links segments of the same class. We present the first multi-class video co-segmentation evaluation. We show that our method is applicable to real video data from online resources and outperforms state-of-the-art video segmentation and image co-segmentation baselines.
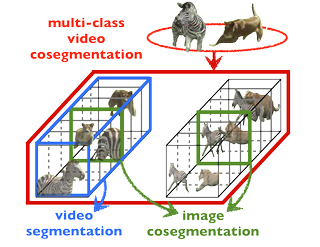
Figure 1. Our proposed multi-class video co-segmentation model addresses segmentation of multiple object clases across multiple videos. The segments are linked within and across videos via the global object classes.
Source code, Dataset
The source code and the dataset can be downloaded here.

References
[1] Multi-Class Video Co-Segmentation with a Generative Multi-Video Model, Wei-Chen Chiu and Mario Fritz, IEEE Conference on Computer Vision and Pattern Recognition (CVPR), (2013)