Video Object Segmentation with Language Referring Expressions
Anna Khoreva, Anna Rohrbach, and Bernt Schiele
Most state-of-the-art semi-supervised video object segmentation methods rely on a pixel-accurate mask of a target object provided for the first frame of a video. However, obtaining a detailed segmentation mask is expensive and time-consuming. In this work we explore an alternative way of identifying a target object, namely by employing language referring expressions. Besides being a more practical and natural way of pointing out a target object, using language specifications can help to avoid drift as well as make the system more robust to complex dynamics and appearance variations. Leveraging recent advances of language grounding models designed for images, we propose an approach to extend them to video data, ensuring temporally coherent predictions. To evaluate our method we augment the popular video object segmentation benchmarks, DAVIS'16 and DAVIS'17 with language descriptions of target objects. We show that our approach performs on par with the methods which have access to a pixel-level mask of the target object on DAVIS'16 and is competitive to methods using scribbles on the challenging DAVIS'17 dataset.

Approach
Given a video with a textual query of the target object, our aim is to obtain a pixel-level segmentation mask of the target object in every frame that it appears. We leverage recent advances in grounding referring expressions in images and pixel-level segmentation in videos.
Our method consists of two main steps. Using as input the textual query provided by the user, we first generate target object bounding box proposals for every frame of the video by exploiting referring expression grounding models, designed for images only. Applying these models off-the-shelf results in temporally inconsistent and jittery box predictions. Therefore, to mitigate this issue and make them more applicable for video data, we next employ temporal consistency, which enforces bounding boxes to be coherent across frames. As a second step, using as guidance the obtained box predictions of the target object on every frame of the video we apply a convnet-based pixel-wise segmentation model to recover detailed object masks in each frame.
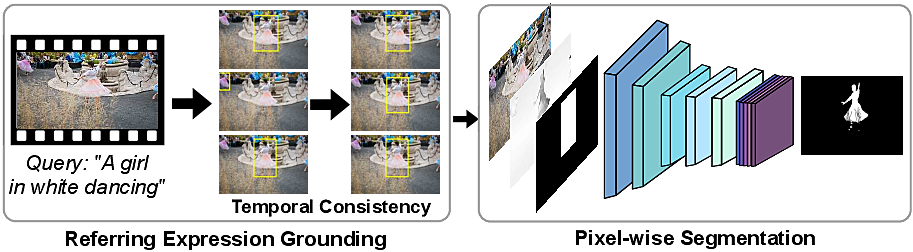
Downloads
Referring expression annotations
For further information or data, please contact Anna Khoreva <khoreva at mpi-inf.mpg.de>.
References
When using the referring expression annotations on DAVIS'16 and DAVIS'17, please cite the following publication:
[Khoreva et al., 2018] Video Object Segmentation with Language Referring Expressions, A. Khoreva, A. Rohrbach and B. Schiele, arXiv preprint arXiv:1803.08006, 2018.
@article{Khoreva_arxiv2018, title = {Video Object Segmentation with Language Referring Expressions}, author = {Khoreva, Anna and Rohrbach, Anna and Schiele, Bernt}, year = {2018}, journal = {arxiv: 1803.08006} }